Setting up a CI/CD Pipeline for AWS Fargate using Gitlab CI CD
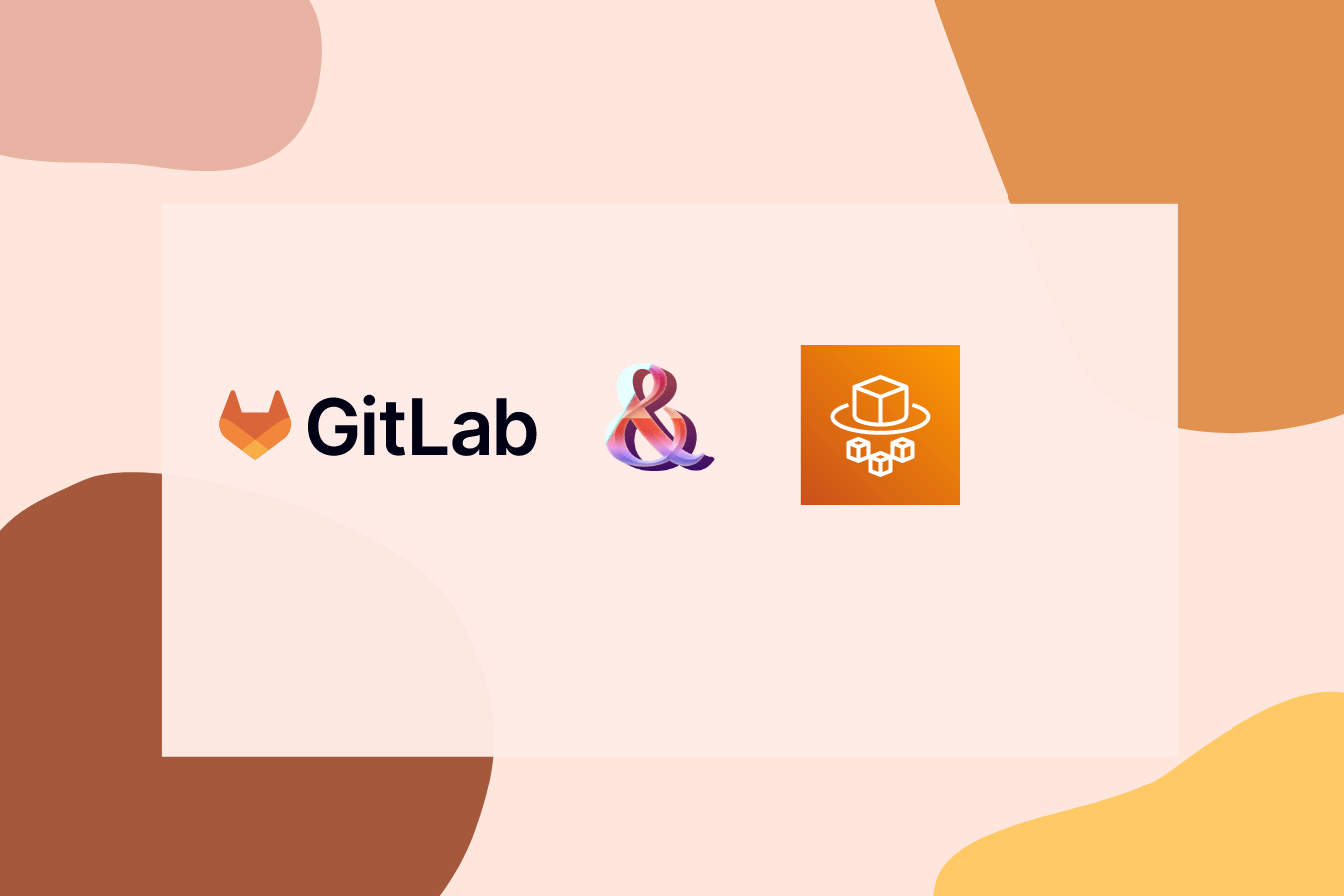
In this guide, you're going to learn how to create infrastructure to host your dockerized application in AWS Fargate using Gitlab CI CD. Then, we're going to learn about how to setup CI/CD pipeline - so that when you push changes to your application - Gitlab CI/CD pipeline will kick in, builds your image and push the image to ECR and then, your fargate tasks will be updated with latest image that has pushed to ECR.
Infrastructure for AWS Fargate:
Below is the high-level architecture diagram for hosting our dockerized application in AWS Fargate.
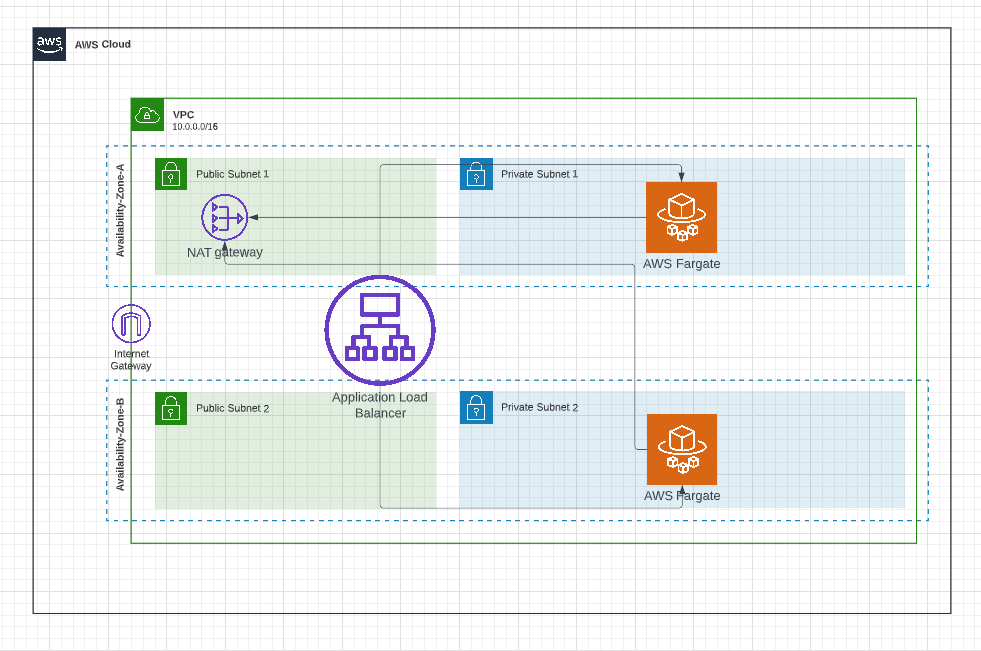
VPC & Subnets:
It is always recommended to have separate VPC to host your application so that you can have logical separation between your application and your other AWS resources and this makes easier to manage. We're going to create a new VPC and we're going to use 2 availability zones to provide redundancy for your application. We're going to create 2 subnets (public subnet and private subnet) in each of these 2 availability zones. So, we're going to create 4 subnets across 2 availability zones.
Application load balancer & Fargate tasks:
We're going to host our fargate application in private subnets so that no one would be able to directly access our application. This provides additional security to our application. Then, we're going to create application load balancer
and this application load balancer
will reside in public subnets and the role of this application load balancer is to route requests from users to our fargate tasks in private subnets.
Nat Gateway:
As mentioned earlier, our fargate app is hosted in private subnets. Private subnets are subnets without any internet connectivity and this poses a problem. When you don't have internet connectivity, how we're going to pull the docker image and additional packages that are required for our application. NAT Gateway
solves this problem. From our private subnet, using NAT gateway, you can access internet to from private subnet for outbound traffic and still all inbound traffic will be blocked.
Let us create this architecture using AWS CDK - an Infrastructure as Code (IaC) tool - developed and maintained by AWS. If you're new to AWS CDK - I strongly suggest you to read this guide to understand AWS CDK. No problem, I can wait.
You can execute below command to create AWS CDK app
mkdir aws-cdk-fargate
cd aws-cdk-fargate
cdk init app --language=typescript
CDK app would have created a stack file in lib
folder.
VPC creation:
Now, we're going to create a VPC resource in this stack.
const vpc = new ec2.Vpc(this, "FargateNodeJsVpc", {
maxAzs: 2,
natGateways: 1,
subnetConfiguration: [
{
cidrMask: 24,
name: "ingress",
subnetType: ec2.SubnetType.PUBLIC,
},
{
cidrMask: 24,
name: "application",
subnetType: ec2.SubnetType.PRIVATE_WITH_NAT,
},
],
});
Above construct will create VPC with 2 availability zones and one NAT Gateway
. In subnet configuration, we're asking CDK to create two types of subnets - public
and private
. Please note that this will automatically create Internet Gateway
as the public subnet requires it. The private subnet will be attached to NAT Gateway as we've mentioned the subnet type as PRIVATE_WITH_NAT
This construct will create 4 subnets - 2 subnets in each of the availability zones and updates the route table
to route traffic accordingly.
Application load balancer creation:
We can create Application Load Balancer
using below construct. The application load balancer is to be associated with public subnets.
const loadbalancer = new ApplicationLoadBalancer(this, "lb", {
vpc,
internetFacing: true,
vpcSubnets: vpc.selectSubnets({
subnetType: ec2.SubnetType.PUBLIC,
}),
});
Cluster creation:
You can use below construct code to create Fargate cluster. We're associating this cluster to the VPC that we've created earlier.
const cluster = new ecs.Cluster(this, "Cluster", {
vpc,
clusterName: "fargate-node-cluster",
});
Execution role:
The ECS agent should be able to pull images from ECR to update your tasks. This permission is represented by execution role
. We're going to create this execution role with predefined managed policy AmazonECSTaskExecutionRolePolicy
which would be used by fargate to pull the image or to log to cloudwatch.
const executionRole = new iam.Role(this, "ExecutionRole", {
assumedBy: new iam.ServicePrincipal("ecs-tasks.amazonaws.com"),
managedPolicies: [
ManagedPolicy.fromAwsManagedPolicyName(
"service-role/AmazonECSTaskExecutionRolePolicy"
),
],
});
Creating ECR Repository:
You need to create ECR repository to store images. A ECR repository is created using below construct.
const repo = new ecr.Repository(this, "Repo", {
repositoryName: "fargate-nodejs-app",
});
Please note that this ECR repository would be used in our CI/CD pipeline to push our images
Creating Fargate service:
We're going to use ecs_patterns.ApplicationLoadBalancedFargateService
construct to create Fargate service - as shown below
new ecs_patterns.ApplicationLoadBalancedFargateService(
this,
"FargateNodeService",
{
cluster,
taskImageOptions: {
image: ecs.ContainerImage.fromRegistry("amazon/amazon-ecs-sample"),
containerName: "nodejs-app-container",
family: "fargate-node-task-defn",
containerPort: 80,
executionRole,
},
cpu: 256,
memoryLimitMiB: 512,
desiredCount: 2,
serviceName: "fargate-node-service",
taskSubnets: vpc.selectSubnets({
subnetType: ec2.SubnetType.PRIVATE_WITH_NAT,
}),
loadBalancer: loadbalancer,
}
);
cluster
: This property tells AWS to use the specified cluster in which fargate service has to be created
taskImageOptions
: The properties specified here would be used to create task definition for your fargate tasks.
image
: This is the image that would be used to create container in fargate. Please note that we're not using our application image. Instead, we're using amazon/amazon-ecs-sample
provided by AWS - This is a simple dockerized PHP application. To create and run fargate service, we need an image. Later, in CI/CD pipeline, we'll be overwriting this image with our custom application image.
family
: This property represents name of the task definition. Any further updates to the task definition will increment the revision number associated with the task definition with the same family value. This family
property would be used in our CI/CD pipeline to update the image.
containerPort
: This is the port exposed by container. As we're going to build a simple web application with HTTP, we're using port 80.
executionRole
: This is the role required by ECS agent which is used for pulling images from ECR and to log to cloudwatch
cpu
: This value represents how much CPU you need for the task. As I'm going to create simple API with couple of endpoints, I've used the value 256 which represents .25 vCPU
. You can increase the value as per your computational needs.
memoryLimitMib
: This value represents how much memory you need for task. I've used 512.
desiredCount
: This is the property which dictates how many tasks that you want to run as part of this service. I want to create 2 tasks just to have redundancy - each task would be created in private subnet of each availability zone. If you're expecting huge usage for your application, you may have to increase this value.
taskSubnets
: This property tell AWS ECS fargate to launch in which subnets. For security reasons, we're going to use private subnets of our VPC created earlier.
loadBalancer
: Represents the load balancer that would be used in our service.
Here is the complete CDK code for creating fargate service with application load balancer
export class AwsCdkFargateStack extends Stack {
constructor(scope: Construct, id: string, props?: StackProps) {
super(scope, id, props);
const vpc = new ec2.Vpc(this, "FargateNodeJsVpc", {
maxAzs: 2,
natGateways: 1,
subnetConfiguration: [
{
cidrMask: 24,
name: "ingress",
subnetType: ec2.SubnetType.PUBLIC,
},
{
cidrMask: 24,
name: "application",
subnetType: ec2.SubnetType.PRIVATE_WITH_NAT,
},
],
});
const loadbalancer = new ApplicationLoadBalancer(this, "lb", {
vpc,
internetFacing: true,
vpcSubnets: vpc.selectSubnets({
subnetType: ec2.SubnetType.PUBLIC,
}),
});
const cluster = new ecs.Cluster(this, "Cluster", {
vpc,
clusterName: "fargate-node-cluster",
});
const repo = new ecr.Repository(this, "Repo", {
repositoryName: "fargate-nodejs-app",
});
const executionRole = new iam.Role(this, "ExecutionRole", {
assumedBy: new iam.ServicePrincipal("ecs-tasks.amazonaws.com"),
managedPolicies: [
ManagedPolicy.fromAwsManagedPolicyName(
"service-role/AmazonECSTaskExecutionRolePolicy"
),
],
});
new ecs_patterns.ApplicationLoadBalancedFargateService(
this,
"FargateNodeService",
{
cluster,
taskImageOptions: {
image: ecs.ContainerImage.fromRegistry("amazon/amazon-ecs-sample"),
containerName: "nodejs-app-container",
family: "fargate-node-task-defn",
containerPort: 80,
executionRole,
},
cpu: 256,
memoryLimitMiB: 512,
desiredCount: 2,
serviceName: "fargate-node-service",
taskSubnets: vpc.selectSubnets({
subnetType: ec2.SubnetType.PRIVATE_WITH_NAT,
}),
loadBalancer: loadbalancer,
}
);
}
}
When you run cdk deploy
to deploy the stack, all AWS resources would be created and the URL for application load balancer would be printed in the console. When you access the URL, you'll be able to see following page
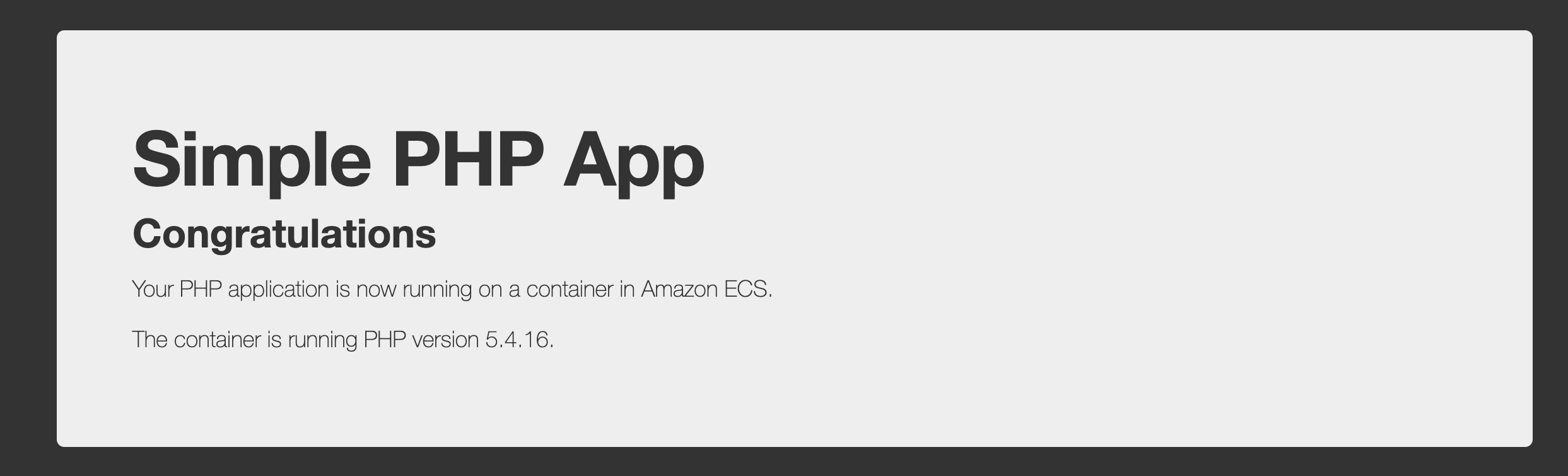
Please note that this is just a sample app. In the next step, we're going to deploy our nodejs app.
CI/CD Pipeline:
If you're new to Gitlab CI/CD, I strongly recommend you to read this guide on Gitlab CI/CD. I'll wait. Please read that guide first. I can wait :-)
Before creating CI/CD pipeline, we're going to create a simple NodeJS app using express.
Simple NodeJS API
const express = require('express');
const app = express();
const PORT = process.env.PORT || 80;
const products = [
{id: 1, name: "Product 1", price: 100},
{id: 2, name: "Product 2", price: 200},
{id: 3, name: "Product 3", price: 300},
];
app.get("/health", (req,res ) => {
res.status(200).send({data: "OK"});
});
app.get("/", (req,res ) => {
res.status(200).send({data: products});
});
app.listen( PORT, () => {
console.log(`Listening at port:${PORT}`)
});
As the objective of this guide is to deploy our nodejs application into ECS service with launch type as fargate - I've made this application to be pretty simple. It just has couple of endpoints - /
endpoint to return list of products and /health
endpoint to return health status of this API.
AWS ECS service requires an image - so we need to create Dockerfile for creating an image out of this nodejs application.
Dockerfile for NodeJS API:
FROM node:16-alpine
WORKDIR /usr/src/app
COPY package*.json ./
RUN npm install
COPY . .
EXPOSE 80
CMD [ "node", "app.js" ]
I've made this Dockerfile simple as our focus is on building CI/CD pipeline for our ECS service.
Below are the high level steps that we need to follow in our CI/CD pipeline using Gitlab CI CD to deploy dockerized nodejs application into AWS ECS service(with Fargate as launch type)
CI/CD variables in Gitlab project repository:
We need to create following CI/CD variables in your project's gitlab repository as shown below. These values would be used in our CI/CD pipeline scripts.
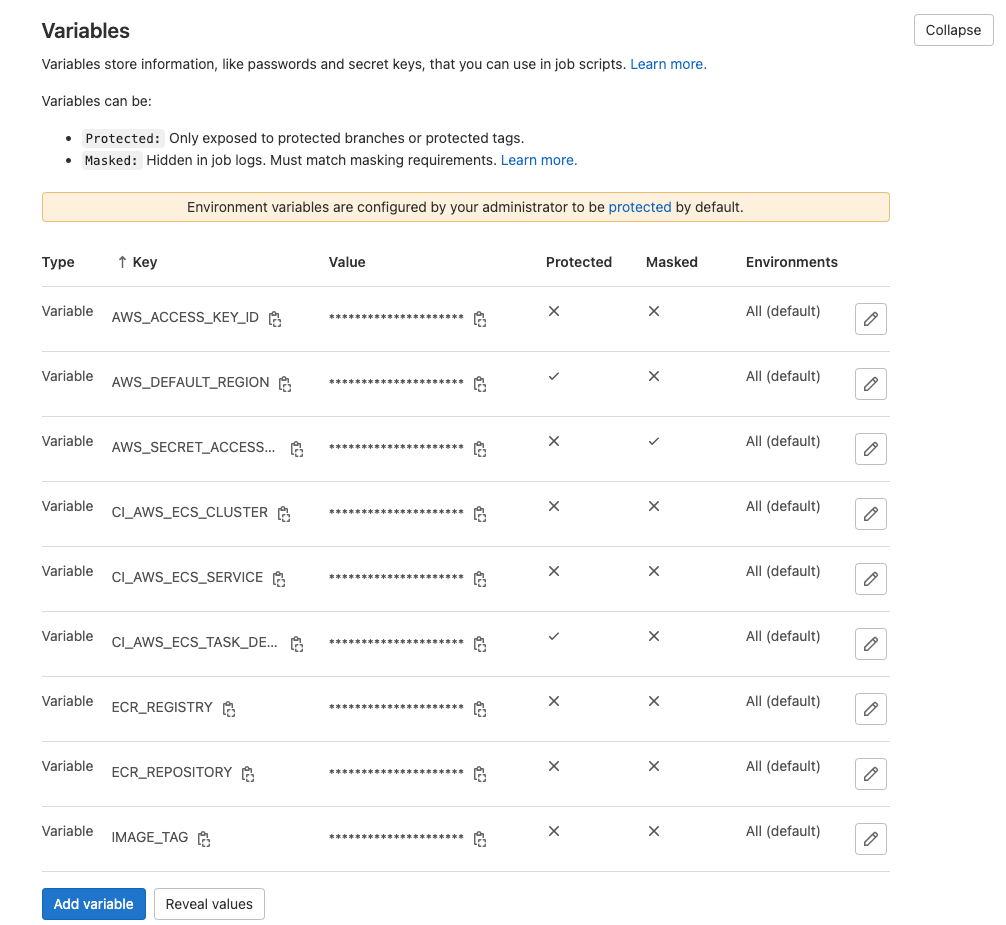
AWS_ACCESS_KEY_ID
& AWS_SECRET_ACCESS_KEY
: These access keys is required to communicate to AWS. Please note that these variable names should be as mentioned above as per AWS CLI expectations.
AWS_DEFAULT_REGION
: This is the default region that AWS uses to execute your commands. Please note that this variable name should be as mentioned above as per AWS CLI expectations.
Below variables are used in CI/CD pipeline and you can name these variables however you want but make sure to update the variable names in script if you're changing it here.
CI_AWS_ECS_CLUSTER
: This is the name of our AWS ECS cluster. While creating the infrastructure for the application - we have given a name fargate-node-cluster
to the cluster.
CI_AWS_ECS_SERVICE
: Name of fargate service. While creating the infrastructure for the application - we have given a name fargate-node-service
CI_AWS_ECS_TASK_DEFINITION
: Name of the task definition. While creating the infrastructure for the application - we have given a name fargate-node-task-defn
ECR_REGISTRY
: Name of the ECR registry. It will be of pattern
<your-account-number>.dkr.ecr.<your-region>.amazonaws.com
ECR_REPOSITORY
: Name of the ECR repository - fargate-nodejs-app
in our case.
IMAGE_TAG
: As the name implies, this is the name of the tag. You can use either the same tag ( latest
for example) or you can different tags based on the commit.
Gitlab CI/CD pipeline for AWS Fargate:
At high level, we're going to have below 2 stages in this Gitlab CI/CD pipeline
- Publish the image to ECR
- Deploy to ECS Fargate
Publish
stage:
Below are the high level steps in publish
stage
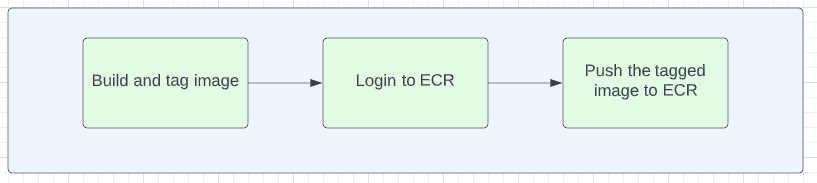
We want to build and tag image based on the Dockerfile in our repository and then we push the image to ECR.
Below is the code snippet for publish stage
variables:
DOCKER_HOST: tcp://docker:2375
publish-to-ecr:
stage: publish
image:
name: amazon/aws-cli
entrypoint: [""]
services:
- docker:dind
before_script:
- amazon-linux-extras install docker
script:
- docker build -t $ECR_REGISTRY/$ECR_REPOSITORY:latest .
- aws ecr get-login-password | docker login --username AWS --password-stdin $ECR_REGISTRY
- docker push $ECR_REGISTRY/$ECR_REPOSITORY:latest
image
section:
We're using image amazon/aws-cli
as we want to execute aws cli commands(aws ecr commands in our case) in our script. It is important to note here is that the entire Gitlab CI/CD pipeline runs inside a docker container.
services
section:
This services
section is for using any additional services. As you know our gitlab CI-CD pipeline is running inside a docker container. But, we need to run docker daemon inside this existing docker container for building our image. For this we need to do 2 steps
- Use the
docker in docker
service - docker:dind - Communicate with existing docker with this new
docker:dind
service. This is done by using a variable DOCKER_HOST as mentioned in the second line of the above script.
before_script
section:
The image amazon/aws-cli
does not have docker by default. So, we're installing docker
by using below command.
amazon-linux-extras install docker
script
section:
We're building and tagging the image in the first line. In the second line - we're logging into AWS ECR so that we can push into ECR repository. And in the third line, we're pushing the image to ECR.
Deploy
stage:
Below are the high level steps involved in deploy
stage

The process is pretty simple : Get the current task definition and update the task definition with the newly pushed image. Then, register this new task definition and update the service
deploy-to-ecs:
stage: deploy
image:
name: amazon/aws-cli
entrypoint: [""]
script:
- aws ecs describe-task-definition --task-definition $CI_AWS_ECS_TASK_DEFINITION --output json --query taskDefinition > task-definition.json
- python scripts/replace-container-defn-img.py task-definition.json $ECR_REGISTRY/$ECR_REPOSITORY:latest
- aws ecs register-task-definition --cli-input-json file://task-definition.json
- aws ecs update-service --cluster $CI_AWS_ECS_CLUSTER --service $CI_AWS_ECS_SERVICE --task-definition $CI_AWS_ECS_TASK_DEFINITION --force-new-deployment
stage
:
This deploy-to-ecs
job belongs to the stage deploy
image
:
As we need to run aws cli commands, we need to have an image with AWS CLI tools installed and hence we're using amazon/aws-cli
image.
script
:
In the first step of the script - we're getting the task definition and store it in local file task-definition.json
. In the second step, we're replacing existing container image with newer image that we've built in previous job. This is a simple python script to replace value of image in task definition. In the third step, we're registering new task definition with the updated task definition that we've created in 2nd step. In last step, we're updating the service with this new task definition - which will replace the existing task using rolling update.
Python script to replace image value in task definition file:
This python script just has couple of functions
get_updated_task_defn
: This function gets the file name and updated image name as parameters. This function reads the data as json from file and replace the image name in containerDefintions
image filed. As this task definition contains only one container definition, we're replacing the image in that container definition. We're deleting some properties as there is a schema difference between describe-task-definition
and register-task-definition
CLI commands.
update_task_defn
: This function just writes back to the same task-definition.json file
import sys
import json
def get_updated_task_defn(task_defn_file_name, updated_image):
with open(task_defn_file_name, 'r') as f:
data = json.load(f)
data["containerDefinitions"][0]["image"] = updated_image
del data["status"]
del data["registeredAt"]
del data["registeredBy"]
del data["requiresAttributes"]
del data["compatibilities"]
del data["taskDefinitionArn"]
del data["revision"]
return data
def update_task_defn(task_defn_file_name, data):
with open(task_defn_file_name, 'w') as f:
json.dump(data, f, ensure_ascii=False, indent=4)
args = sys.argv[1:]
task_defn_file_name = args[0]
updated_image = args[1]
updated_task_defn = get_updated_task_defn(task_defn_file_name, updated_image)
update_task_defn(task_defn_file_name, updated_task_defn)